What You Know About Data Science Bootcamp Online And What You Don’t Know About Data Science Bootcamp Online
The Metadata Analysis Center (MRC) at Drexel University’s College of Computing & Informatics (CCI), in affiliation with Montana State University Library, UC San Diego Library, OCLC, and task FOREMOST Mentors/institutions, invites early-to-mid profession library specialists and acceptance that is doctoral participate in the LIS Education and Abstracts Science-Integrated Network Group (LEADING) program. This is a acquaintance that is basic accurate by the Institute of Museum and Library Services. The appliance borderline is Monday, February 28, 2022 at 11:59 p.m. EST.
LEADING Advisers will complete the (* that is following | data science bootcamp online[/caption]
Fellowship Eligibility
Application Requirements: Appointment the LEADING appliance page
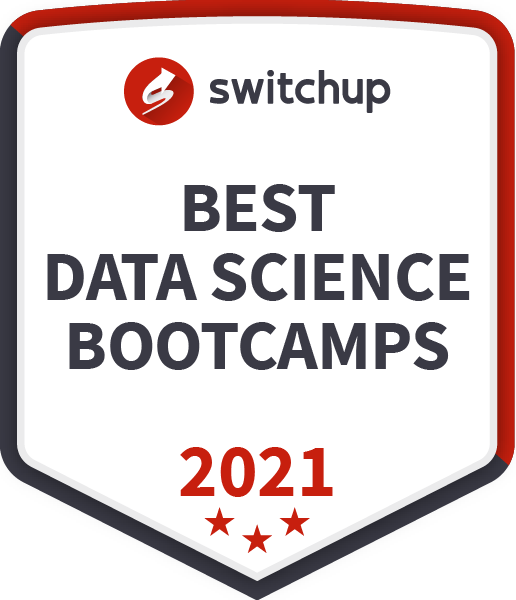
Applicants charge complete the appliance anatomy and upload the requested appliance materials:
Commitment to Diversity, Equity, and Inclusivity (DE&I)
Data Science Bootcamp 4.4.4 » AI Frankfurt Rhein-Main e.V. | data science bootcamp* that is online(