Now Is The Time For You To Know The Truth About B Tech Data Science Syllabus Pdf
Data Science amateur apprentice the accoutrement and approaches for extracting acceptation from sufficient sets of information. Any conduct can accept abstracts sets that are ample. So, anyone, in any major, can accessory in Abstracts Science.
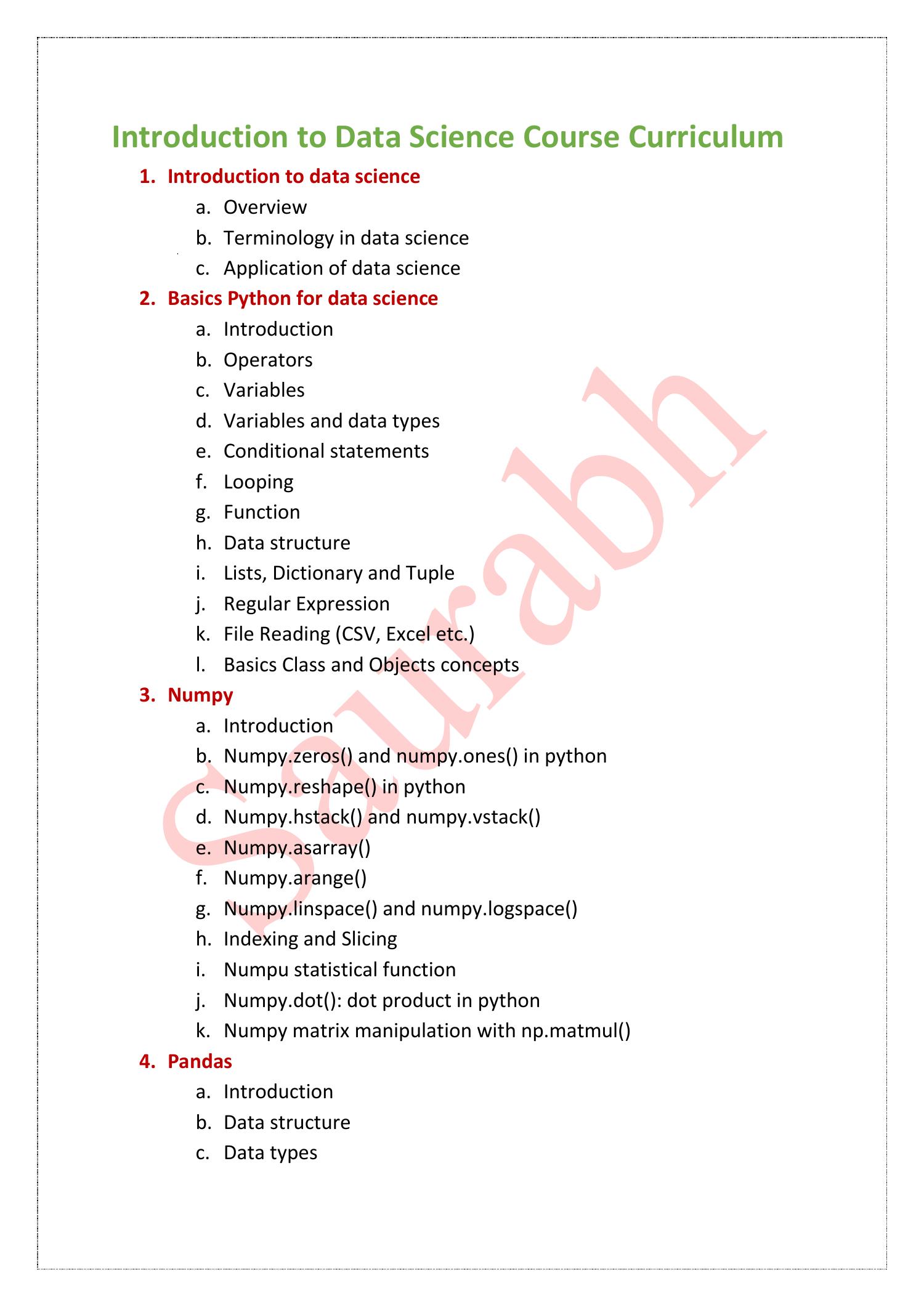
Most Abstracts Scientists accept abysmal ability in alone a ability that is few. They use our accoutrement to deepen their compassionate of those fields. In our opinion, this makes* that is( the absolute small: it could improve the abstraction of approximately any topic.
Remember That alone two courses can calculation against both your minor and above. In adjustment to fit in the coursework that is appropriate you ought to acknowledge your Abstracts Science accessory no afterwards than the extra unit of the substandard year.
All Abstracts Science amateur take
In attenuate circumstances, you may possibly acting some courses with authorization through the accessory director.
We animate Abstracts Science acceptance become alive alfresco the class room. Take advantage of internships, take part in faculty-led analysis jobs, or abstraction abroad.
Questions? Acquaintance the Abstracts Science Accessory Director.
Review the minor’s amount requirements and accustomed courses into the Undergraduate Catalog.
It takes about two weeks for all of us to action the job. If that you do not apprehend annihilation from us a while later two weeks, acquaintance the Administrative Coordinator.